It’s one thing to have data, it’s another thing to consider whether the data that you have, is actually any good.
When I say good, I am of course referring to the characteristics of the data that make it useful in your organization.
It doesn’t matter how big your organization is, if you’re not concerned about your customer master data quality then perhaps it is time to rethink that position and start to worry about it.
You likely don’t realize what your direct competitors are doing about their customer data and if you could find out, you would likely find that they are already working on their customer master data management strategy and data quality management approach.
Implementing data quality practices helps an organization manage and govern customer data more effectively. When customer data quality management is baked into the data acquisitions and change management process across an organization, there is a fast ROI and positive improvement in not just marketing and messaging campaign management and yield but also operational efficiency, data privacy adherence, regulatory compliance, and generalized business decision-making.
What you should know about customer data quality
Customer data quality is a critical piece of your overall customer data management and data governance practice.
Not a one-off
Customer data quality is not a “do-once and you’re done” exercise. It is easy to think it could be if you have just migrated from manual methods or from an old system. Instead, data quality management just like customer data management in general is a continuous process that must be constantly and proactively improved iteratively.
Customer data quality is bound to rules and processes and overseen by your customer data governance practice which encompasses your business policies. The policies are perhaps more abstract but a lightweight approach can see your data quality rules summarise the related business policy.
Compounding
Customer data quality issues have a compounding effect. The smallest of errors in your customer master may present a myriad of issues to downstream functions and processes that would be unforeseen and unable to be predicted. This error or defect in the data can then result in cascading reactions.
When you haven’t established the correct checks and balances for the data, it then becomes difficult to remediate the resulting problems. If the issue is left unresolved it can continue to produce problems that lead to deterioration of operational efficiency and effectiveness, compromise reporting, compromise compliance and negatively impact organizational decision-making.
An optimized customer data governance practice focuses on customer data management and its appropriate data quality control, reporting, and remediation at the earliest stage in the customer data capture process.
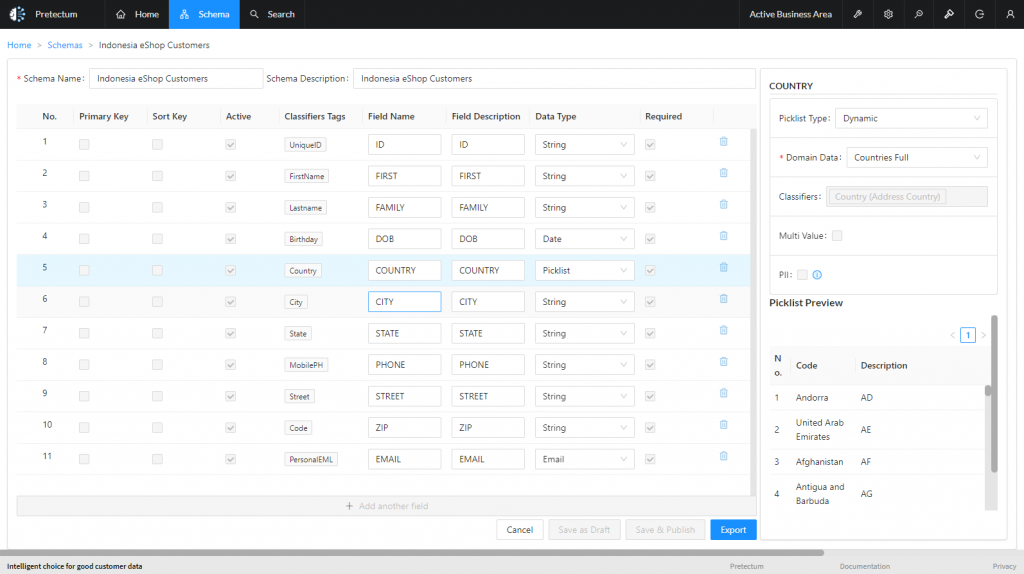
Knowledge is power
A practice of continuous customer data quality management is unachievable if you don’t know where the risks and problems lie.
You need to have defined what good data looks like and established tools or practices to support the evaluation of the data quality. You then need to have taken an inventory of the systems that you have, that contain customer data and then you need to have run the assessment.
With the average selling organization in all likelihood having at least three systems, an order system a shipping system, and an accounting system; that’s three potentially different places to store structured customer data assuming none is to be found in spreadsheets. In some instances, these will be unified in the eCommerce, CRP, or ERP system but then the CDP or marketing tech platform will also likely have customer data too.
From this, we can deduce that the customer master data architecture is fragmented and potentially siloed in a way that prevents the organization from leveraging a unified single customer view.
Data in each source may be slightly different and even conflicting.
Data quality assessment of each system will tell you what the current state is of each of these system’s customer masters.
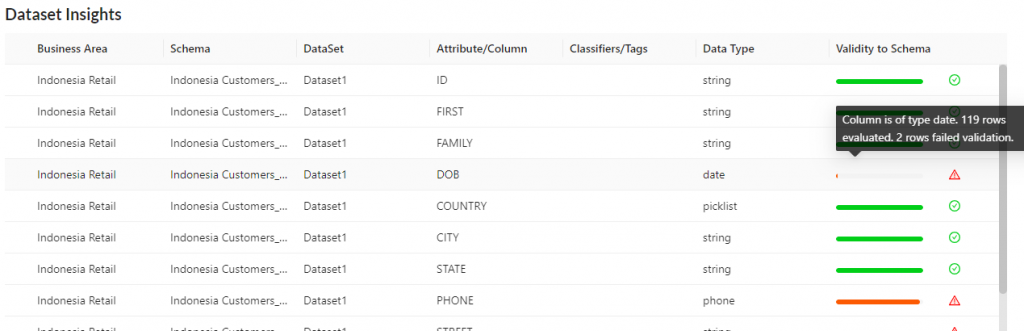
Operational efficiency goes up and costs go down
Resolving data quality introduces data management friction in that it requires someone to identify the issue, identify the root cause, and then work towards the correction. If the decision or choice is made, that no correction will be made, then workarounds need to be put in place.
Manual identification, reconciliation, and correction are inefficient, slow, and expensive and tie up resources that would be better assigned to higher-value work.
When a comprehensive customer data quality approach is adopted that includes continuous data quality checking at the time of capture and is supported by regularized reporting, an organization that does this not only reduces errors but also suppresses the fire drills and manual investigations accompanied by the wasteful effort of repeated activity.
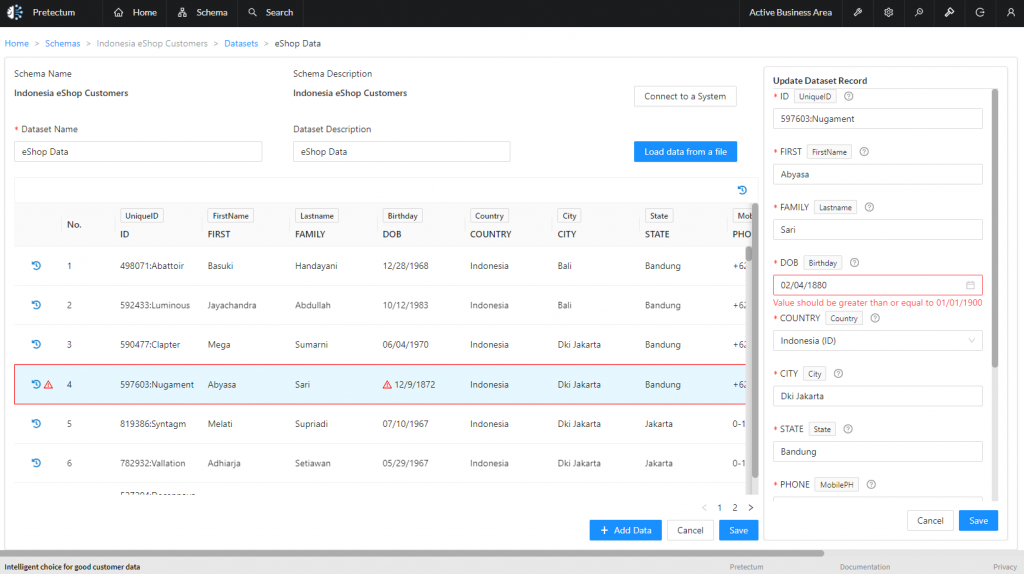
Bad data can get you into trouble
Even if your organization is not in a regulated industry, the reality is that the moment you start storing customer data, you fall under the potential scrutiny of lawmakers and regulators who will focus on whether you need the need, how you acquired it, what you do with it and whether it is accurate.
There’s more rigor in the health and finance industry segments but every organization should take both privacy and data quality seriously when thinking about how they manage their customer master data.
If you’re subjected to a SAR, a compliance check or audit, or any kind of scrutiny, being able to confidently prove that you have the data for legitimate reasons and have the customer consent accompanied by impeccable data quality, then the investigation should go more easily and be less of a concern.
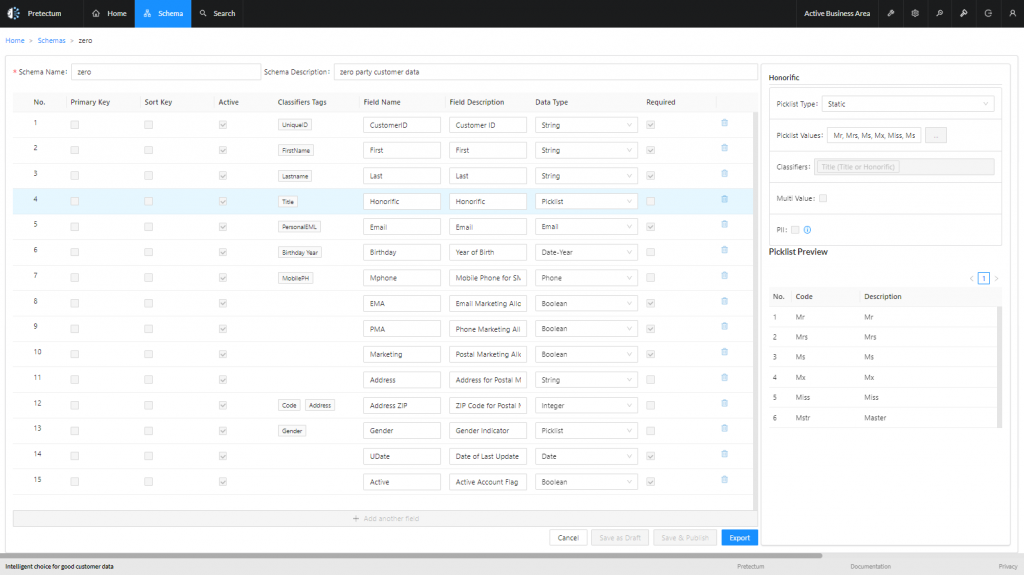
Bad data – bad decision
A bad address is one thing, the customer doesn’t get what they ordered in a timely fashion, and an invoice doesn’t get delivered and promptly paid. These are the kinds of things that organizations have labored with the burden of, for many years.
Apart from the risk to your organization’s reputation, these carry the additional costs of misdelivery. Another aspect that is characteristic of bad data is that you may be using that data to make not just tactical decisions but strategic ones too.
Low-quality business data in the customer master results in inaccurate customer insights. This in turn results in leaders making misinformed decisions. Some of the downstream implications are knowledge about consumer spending power, consumer behavior, consumer interests, and of course all essential contact data.
With continuous data quality in relation to the customer master, your firm can make better-informed decisions, and then monetize that data for direct top-line growth and increased profits by building plans around the data and using it for personalization and improved customer experiences when you engage with them.
Data quality and data governance platforms don’t suit all of us
There are a number of data quality and data management solutions on the market, some of which are suitable for small and medium-size organizations but most of these solutions tend to approach customer data management passively and are batch based.
There are two ways to think about data governance. An approach that is either active or passive.
A passive approach tends to be fix-after-the-fact and an active approach focuses on all stages of the data management lifecycle.
If they are dual mode – batch and realtime, passive and active, then they tend to be very elaborate and very expensive and take a long time to implement.
The Pretectum CMDM is dual mode and is offered as a SaaS customer master data management platform for both batched use and real-time use. Records can be captured in bulk through the UI or singly as records, or they can be maintained via API.
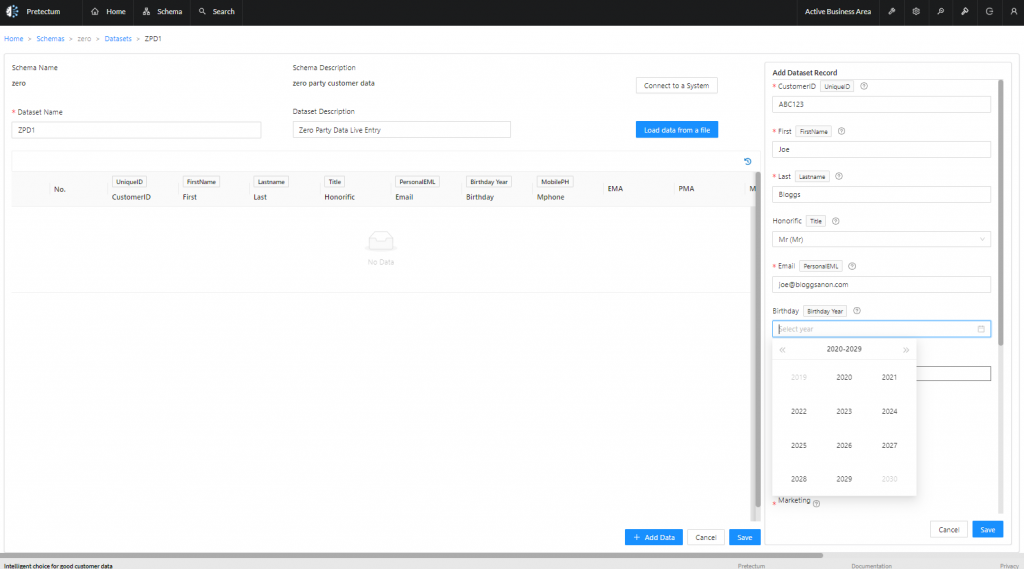
Data quality is a business priority that needs to be continuous and refined iteratively within the whole organization. The Pretectum CMDM is a code-free platform offered as a SaaS solution , specifically for business users but useful to IT, which can be easily accessed via a modern UI and integrated via APIs for use when systems integration is required.
With the Pretectum CMDM platform, your organization gets access to governed customer master data of the highest possible quality, this, in turn, improves your operational efficiency and effectiveness and helps with meeting your compliance obligations while supporting improved decision-making.
Contact us today to learn how you can implement an active customer master data management program today.