Master Data Management (MDM) has gone from an auxiliary data management service adopted by only the largest and most complex of organizations and transformed itself into program-critical functionality for SMBs too.
Many organizations now consider MDM as integral to their success. This is no less true for Customer MDM (CMDM) in particular. Having a dismissive perspective concerning CMDM and regarding master data management altogether as an expensive, time-consuming, and patience-testing trivial pursuit is, for many, long gone.
Today, CMDM is recognized as more than just practice and more than software; it is now a comprehensive initiative that plays a definitive role in ensuring compliance and customer data hygiene. Underpinnings include improved data reliability, completeness, and readiness of customer master data for insightful decision-making and business action.
In the contemporary business landscape, where data is a cornerstone of operations, CMDM is a highly crucial discipline. Business and IT converge collaboratively in their work to achieve customer data uniformity, accuracy, stewardship, semantic consistency, and appropriate accountability of the shared customer master data asset. The significance of CMDM in organizational processes is the bedrock for informed decision-making and efficient business operations.
As organizations embark on various digital transformation journeys, CMDM is being recognized as a core element in the evolution. The future of CMDM practice is shaped by organizations’ ability to leverage SaaS technologies in particular. These take advantage of Big Data, Large Language Models (LLM) Machine Learning (ML), and Artificial Intelligence (AI) and play pivotal roles in shaping next-generation CMDM. Such technologies offer unprecedented opportunities for organizations to enhance data management capabilities, and drive efficiency, accuracy, and strategic insights.

Data Management for Data Lakes:
The traditional approach to data warehousing (DW) is mostly obsolete, DW is now displaced by data lakes and data virtualization platforms. Data lakes, in contrast to DWs, store vast amounts of data, often in native formats as raw, unstructured bits and bytes. This provides flexibility and scalability.
Designing and implementing a data lake is however a complex undertaking. Without proper transformation, loading data from legacy systems into such platforms can lead to chaos.
The solution lies in effective Metadata Management and Data Governance to extract true value via Big Data Analytics. A precise CMDM strategy is imperative for ensuring data lakes serve as valuable sources, transition, and target assets rather than devolving into unmanageable data quagmires.
Interactive De-duplication using Active Learning:
De-duplication of customer records is a core functionality of CMDM tooling like the Pretectum CMDM. In the past, one would rely on manually created matching rules and policies. The complexity of these often hinders efficient decision-making, and the consequences of changes can be challenging to predict across large data in particular.
Machine Learning Models offer a solution by training on smaller subsets with affirming or refuting labels and those are then applied as machine learning training to larger datasets. Such models not only predict record matches but also assist in optimizing record match policies. The incorporation of ML in de-duplication processes enhances accuracy and efficiency, laying the foundation for more robust CMDM practices.
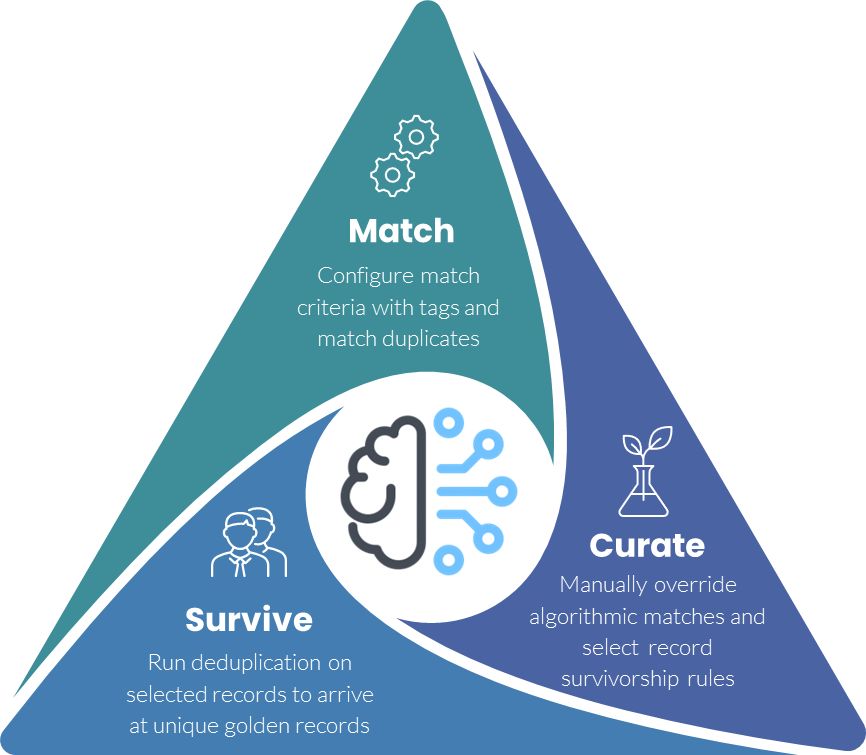
Automated Data Stewardship using Machine Learning:
The past decade has also borne witness to a shift from “data-aware” information systems to “process-aware” systems, such as Workflow Management Systems.
Data Governance and Workflows support routing work items to human supervisors, existing legacy workflows often lack optimization based on real-life execution timelines.
Machine Learning has the potential to revolutionize these Data Governance workflows by training and optimizing processes based on learnings from actual behavior around data. Such optimizations hold the potential for reducing the time spent on improving process efficiency, allowing Data Stewards to focus on more productive tasks during high-volume critical seasonal fluctuations, ultimately enhancing overall operational efficiency.
Pulling out a crystal ball is always challenging, few would have expected the stratospheric rise of LLMs in 2023, so we need to acknowledge that the technology is evolving rapidly but still anticipate the trajectory of CMDM in the coming years.
Empowering the customer
Providing customers with control and transparency over their data. By implementing permission-based strategies and user-friendly preference centers, organizations enable customers to dictate how their information is used.
This shift towards permission-based data management fosters a more open dialogue, allowing customers to set preferences and interests, enhancing their digital experience. The evolution towards customer control aligns with the changing landscape of data regulations, emphasizing the ethical collection of first-party and zero-party data. In this empowered environment, customers not only contribute to more relevant interactions but also actively shape their digital engagement, creating a mutually beneficial and trusting relationship with organizations.
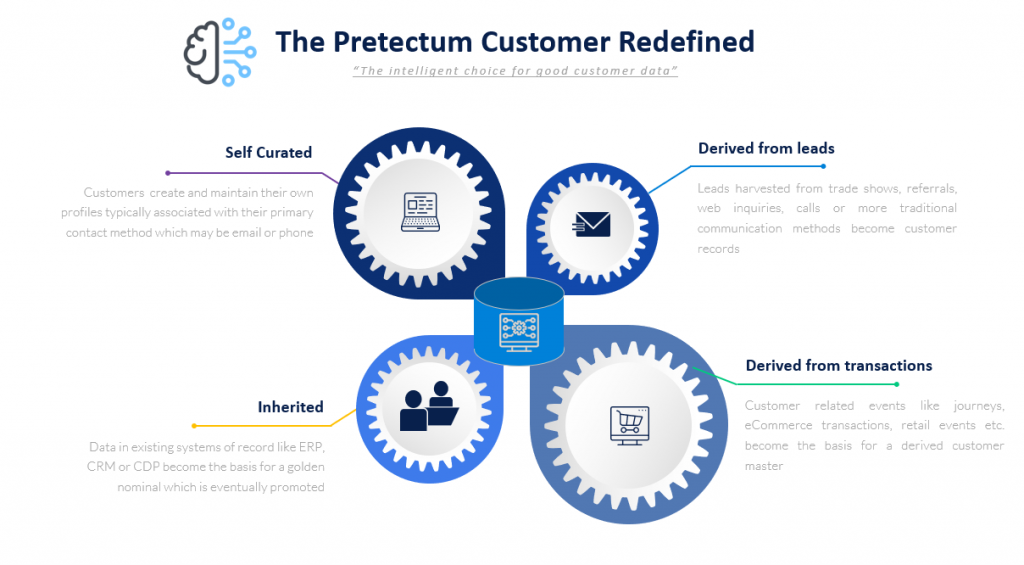
Leveraging the Power of Big Data, LLM, ML, and AI
The integration of Big Data, Machine Learning (ML), and Artificial Intelligence (AI), along with Large Language Models (LLMs), will continue to break ground in efforts around better Customer Master Data Management.
Leveraging these advanced technologies will allow organizations to extract actionable insights, enhance data accuracy, and streamline CMDM processes. Big Data will facilitate the storage and analysis of vast data, while ML and AI optimize de-duplication, matching, anonymization, synthesizing, and customer data protection, all in favor of more efficient data stewardship accompanied by unparalleled precision.
LLMs contribute to natural language processing, enabling a deeper understanding of customer preferences. This technological synergy empowers CMDM to evolve dynamically, meeting the challenges of data complexity, ensuring compliance, and delivering a personalized and responsive customer experience in the ever-evolving digital landscape.
Data Management for Data Lakes and DW
CMDM acts as a crucial defense against data chaos in both data lakes and warehouses. In data lakes, CMDM ensures the precise ingestion of relevant customer data, employing Metadata Management and Data Governance strategies to avoid swamps caused by uncontrolled data accumulation.
In traditional warehouses, CMDM’s emphasis on accuracy and cleanliness prevents the creation of complex and tangled datasets, reducing the risk of quagmires. By instilling discipline in data management, CMDM proactively safeguards against the emergence of unmanageable or low-quality data, enhancing the efficiency and reliability of both data lakes and warehouses. Its targeted approach ensures that these repositories remain structured, well-governed, and conducive to effective analytics.
Holistic Integration
CMDM is also poised to revolutionize digital transformation by ensuring holistic integration with heterogeneous systems that store and utilize customer data.
As organizations evolve digitally, CMDM becomes the linchpin in unifying diverse data sources. Its impact extends beyond data accuracy and governance, influencing seamless interoperability across systems.
CMDM systems’ structured approach harmonizes customer data, facilitating a Single Customer View, a critical element in enhancing customer experiences. Through precise integration with various systems, CMDM enables organizations to break down silos, providing a comprehensive understanding of customers. This transformative role positions CMDM as a catalyst for streamlined operations, informed decision-making, and ultimately, the success of digital initiatives by creating a unified and enriched customer data ecosystem.
Alignment with Business Goals
CMDM is essential in aligning business goals and fostering value chains that businesses can derive substantial benefits from. By ensuring the accuracy and uniformity of customer data, CMDM becomes integral in supporting strategic business objectives.
This role extends beyond data governance, influencing the creation of interconnected value chains. CMDM systems facilitate a more comprehensive understanding of the customer, thereby enabling businesses to tailor products and services, enhance customer experiences, and drive revenue growth.
This cohesive approach not only breaks down data silos further but also forms the backbone for informed decision-making. CMDM then becomes instrumental in creating agile and responsive value chains that align seamlessly with business goals, unlocking efficiency, innovation, and sustained success.
As we navigate the dynamic landscape of digital transformation, CMDM will continue to play a pivotal role in ensuring data accuracy, uniformity, and reliability. Organizations that embrace the evolving nature of CMDM, integrate it holistically into their digital strategies, and leverage innovative technologies will be well-positioned to thrive in the data-driven era. The journey of CMDM is an ongoing evolution, marked by continuous innovation, adaptability, and a steadfast commitment to achieving business objectives through effective data management.