The concept of value chains, originally introduced by Michael Porter in the field of strategic management, has meaningful application in the context of customer data management.
While the traditional value chain model encompasses activities from inbound logistics to service, focusing primarily on physical product flows, the essence of value creation can be extrapolated to the realm of data without emphasizing the logistical elements.
Consider then, how the principles of value chains apply to data management, examine the stages of the data lifecycle and the parallel philosophy that emerges, and determine for yourself just how much value your customer is, or could be adding to your organization and ultimately customers themselves.
The Data Lifecycle
Data Lifecycle Management (DLM) serves as a comprehensive framework for managing data from its inception to its eventual retirement. In the absence of logistical considerations, the Data Lifecycle emphasizes the holistic progression of data through various stages, each contributing to the overall value derived from data assets.
Similar to the inbound logistics phase of the value chain, data creation marks the genesis of value in the Data Lifecycle. At this stage, raw data is sourced from diverse channels, such as sensors, databases, and external feeds. The quality and relevance of data at its creation lay the foundation for subsequent value extraction.
In the operational phase, akin to operations and outbound logistics in the value chain, data undergoes ingestion, processing, and storage. This stage involves transforming raw data into a structured format, cleaning inconsistencies, and storing it in repositories like data lakes or databases or the case of customer master data, in a customer master data management (CMDM) system or customer data platform (CDP). The efficiency of these operations significantly influences the overall effectiveness of the data management practice.
Drawing parallels with marketing and sales activities in the value chain, data processing and analysis represents the extraction of value from raw information; business data-based techniques such as analytics, machine learning (ML), and statistical modeling uncover patterns, trends, and insights, turning data into actionable intelligence. Pretectum CMDM mostly undertakes this as part of the synchronization process with sources but it also bears fruit in the data quality, deduplication and profiling process stages.
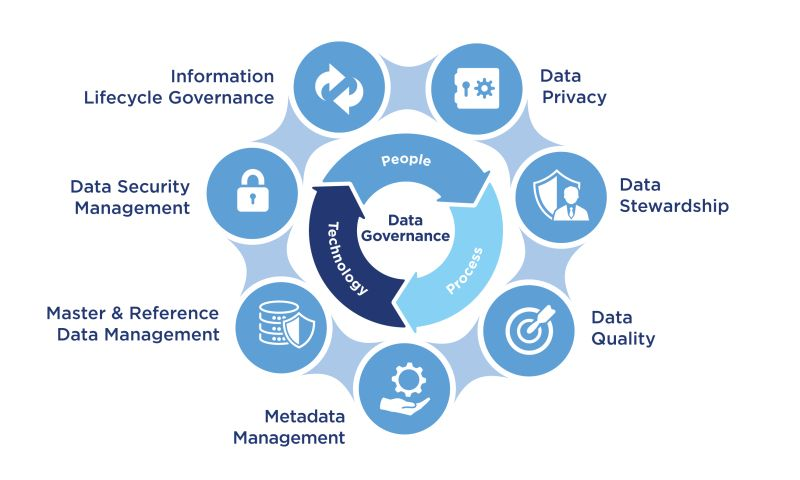
Comparable to the service phase in the value chain, data distribution, or what we refer to as syndication, involves sharing analyzed insights or the finished product with system end-users, decision-makers, or other systems. Whether through reports, dashboards, or real-time data feeds, this phase ensures that the value extracted from data is effectively disseminated across the organization to best effect.
In data management activities, there are practices related to data archiving and retention that align with the procurement and infrastructure aspects of the value chain. This stage ensures that valuable data is appropriately stored, secured, and available for future use, contributing to the overall data governance strategy.
Similar to human resource management in the value chain, data governance and compliance focus on establishing policies and practices to ensure data quality, security, and regulatory adherence. This stage is crucial for maintaining the integrity and reliability of data assets. In this vein, you can consider how you control the distribution and access to data and how you establish your role-based access and control policies.
In the service and infrastructure aspects of the value chain, data destruction aligns with the retirement or disposal of data. This critical stage ensures that obsolete or redundant data is securely and ethically eliminated, preventing potential risks associated with retaining unnecessary information.
Beyond the Value Chain
While the Data Lifecycle serves as a structured framework for data management, a parallel philosophy emerges, emphasizing the interconnectedness of various data-related activities. This philosophy extends beyond the linear progression of stages and considers the dynamic relationships between different facets of data management.
Collaboration and Integration opportunities within a value system serve as a parallel philosophy in data management that encourages breaking the typical data management silos that seem so ubiquitous in many organizations and in turn fosters collaboration between different departments and functions. Recognizing the interconnected nature of data and its usage potential allows organizations to derive more comprehensive insights and drive informed decision-making.
Just as the value system considers interactions between multiple firms, the parallel philosophy in data management extends beyond organizational boundaries. Organizations can benefit from understanding how data interacts with partners both internally and externally, data suppliers, and the broader industry ecosystem. This perspective enhances opportunities for collaboration, data sharing, and ecosystem-wide innovation.
Aligning with the strategic use of customer master data in the value chain, the parallel philosophy underscores the importance of leveraging customer data for competitive advantage. Organizations need to identify areas where customer data can contribute to operational efficiency, customer relations, insights, or innovation, then align data strategies with broader business objectives.
Just as the value chain explores opportunities for monetizing physical assets, the parallel philosophy in data management encourages organizations to explore the monetization of data assets. Here we’re not advocating for selling customer data, rather we’re suggesting that the availability of augmented data has a value placed in mind depending on the application. This could involve selling data to external entities through data partnerships, but really it is often about creating value-added services or even developing new revenue streams through innovative use of customer data such as fee-pay loyalty and membership programs.
Mirroring the feedback loops for continuous improvement in the value chain, the parallel philosophy in data management emphasizes the importance of feedback loops. Analyzing data usage, performance metrics, and user feedback allows organizations to iteratively improve data management processes, ensuring ongoing optimization and adaptability.
The principles of value chains from the world of logistics, when applied to customer data management, reveal the possibilities for a parallel philosophy centered on the interconnected stages of DLM. This holistic approach emphasizes not only the sequential progression of data but also the dynamic relationships, collaborations, and strategic considerations that contribute to the overall value derived from data assets.
Recognizing the parallels between the value chain and data management, organizations can develop a more nuanced understanding of how customer master data creates value throughout its lifecycle. This, in turn, enables informed decision-making, efficient resource allocation, and the development of data strategies that align with broader business objectives. In our heavily data-centric world, where information is a critical organizational asset, embracing this parallel philosophy ensures that the full potential of data is harnessed for sustainable competitive advantage and innovation.