Putting a price on data is a tough task to perform if your business is not “in the business” of selling data. The real question though, is does your organization recognize its arguably most valuable data asset – customer master data, as a valuable resource? The true value of that data more than likely hides in how you use it.
Customer data holds enormous economic potential if managed well and appropriately leveraged. The challenge though is that many companies struggle with proper handling and syndication of their data. Further, they don’t think about their customer data strategically. They don’t take what they have and convert the data that is in hand, into actionable information. Worse, they may not be managing their customer data in any meaningful way, they may have data all over the place in systems, spreadsheets and even email.
By implementing some data management best practices around customer data, these same businesses could be thinking more strategically especially if they are ensuring that their data is accurate and aligned with their business objectives.
The practice of data governance has many facets. Master Data Management is just one of those and certainly, your master data management practice doesn’t have to cover all of these, particularly if the maturity of a given organization is not appropriate to an over-investment in policies and procedures, but there are some basics that you could and should consider.
Data governance includes all of the activities relating to the planning, implementation, development, and control of the information generated by an organization.
In other words, data governance is the development and realization of all the aspects that you might consider for data definition, creation, collection, storage, access, quality, change management and data distribution. Data governance also covers both the master and transactional data.
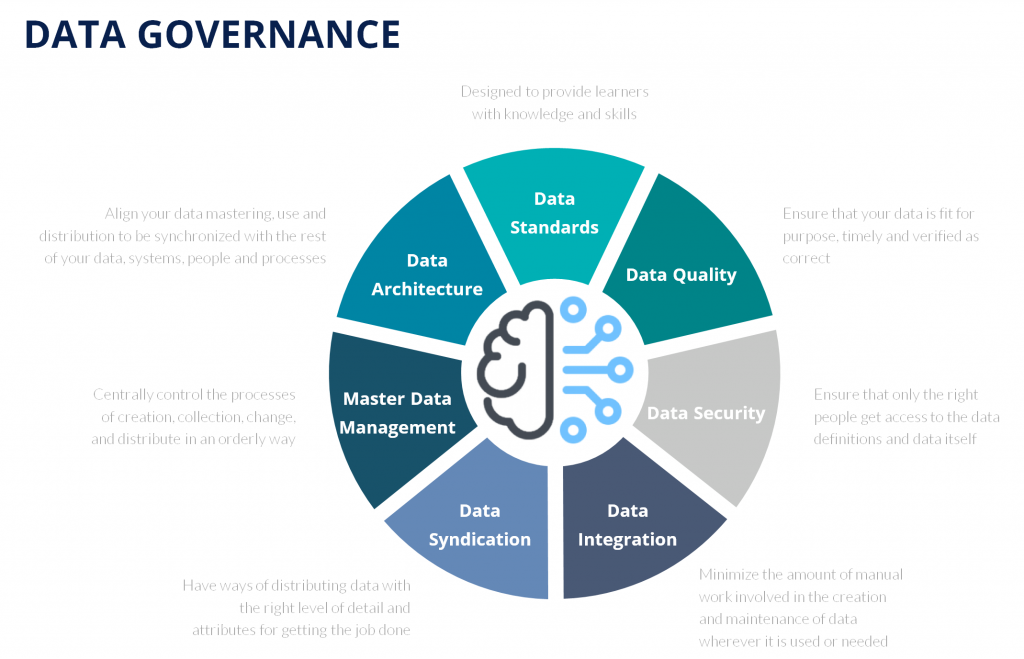
Pretectum sees data governance and the broader discipline of data management as covering many areas including data security, sharing, integration, architecture, MDM (Master Data Management), RDM (Reference Data Management), Insights and Intelligence, change management, storage, retention and retrieval
The best data is the data that is available and usable at exactly the right place and time, in the right format. While that is easily said, achieving this goal is a little more challenging depending on the maturity of your business practices, technology, the characteristics of the data itself and the nature of your industry.
There are key critical strategies and best practices that are generally accepted for improving the way companies manage their data, consider these aspects.
Forget the Rule of Thumb
We use the term frequently, and many businesses actually run their data governance program, if they have one, using the “Rule of Thumb”, but this approach is downright wrong.
At best, “Rule of thumb” is what you might consider an approximate method for data governance, sure, it is based on practical experience, but it is tribal in nature and really only as good as the individuals and the experience that they have with the maintenance of the program.
Having its roots in seventeenth-century trades where weights and measures were almost non-existent and certainly not measured and prescribed, quantities were measured by comparison to the width or length of some sort of loosely defined measure. A thumb, for example often being used as the baseline. These rough swags are fine when you don’t need more control and precision but unfortunately with the growing interest in compliance and meeting regulatory policy, managing data in a loose way is no longer acceptable.
Apart from any business rules you might have in place, there may be a raft of additional rules that are imposed by regulators specifically because of the nature of the data.
Having a robust approach to data governance provides some assurance that data meets the needs of the business and the legal obligations of the environment in which the business operates.
Data governance programs have several hurdles to overcome in order to be part of the DNA of the way the business views and deals with its data. There are cultural challenges, organizational politics and the simple mechanics of basic data control and management.
The technology aspects may be the least of your concerns if you have the right expectations defined in terms of requirements and the right level of complexity and flexibility in a given selected vendor.
The Pretectum CMDM affords you a number of different ways to meet your organizational objectives for Customer Master Data Management as part of a larger data governance program. Reach out today to learn more.